By Eugene Reyes and Jason Boro, with Tina Lieu
Attorneys performing multilingual eDiscovery face the constant challenge of having too much data for humans to review. With particularly large volumes of text, it is prohibitively expensive to translate everything. Rosette and Linguistic Systems Inc. (LSI) tackled that challenge in a legal case and achieved astonishing results. The solution combined the AI-powered text analytics of Rosette by Babel Street with Ai Translate by LSI — a translation solution that leverages AI-based machine translation technology plus expert human translators in an ISO-certified secure environment. (Watch the webinar recording about this project.)
The legal case
The client was a computer chip manufacturer who alleged that an employee stole trade secrets about the product design, testing, improvements, and fabrication of the chips, taking proprietary information to a different manufacturer.
To begin the multilingual eDiscovery process, the client provided case-relevant exemplary emails that contained terms related to chip design, testing, and fabrication — such as alignment, analysis, correction, defect, design, equipment, evaluation, method, processing, reticle, specification, and wafer. The target data collection for eDiscovery included business emails between engineers, written in English and Japanese, about all stages of the company's chip design, manufacturing, and testing procedures.
Traditional multilingual eDiscovery
Often eDiscovery cases — multilingual or not — begin with a search of the data collection using case-relevant keywords. However, keyword search cannot match words unless they are exactly the same — thus “accident” will not find “mishap” or “incident.” Furthermore, not all occurrences of a word are relevant. Based on context, a word can have multiple meanings. “Interest” can refer to “fascination” or “payment on money lent.” Irrelevant results waste attorney time and keyword search alone might only uncover a fraction of relevant documents.
From an English perspective, having to conduct multilingual eDiscovery review with Japanese documents either requires an expensive Japanese-capable attorney or someone who can translate the documents or search queries into Japanese. Human translation of all documents is prohibitively expensive and more affordable machine translation can be patchy and error-ridden. The end result is that keyword search tends to miss substantial amounts of dispositive evidence (meritorious facts that could be relied upon to resolve a legal dispute).
The legal team needed:
- A search method that could access more context than keywords provide
- Better machine translation to enable non-Japanese-speaking attorneys to review relevant culled documents
New paradigm: Natural language processing and machine translation
Keywords fail to provide enough context to filter out irrelevant search results. Sentences provide ample context but are too specific to result in a match. Phrases presented the happy medium between keyword terms and sentences. However, phrases alone might not bring back enough exact matches, so instead of regular keyword search, LSI and Rosette used fuzzy search based on meaning (also known as semantics).
In doing so, they found that phrases were the key to improving machine translation, too.
Finding case-relevant phrases
The system that they built worked as follows. First, Rosette identified the language of each document from the exemplary emails and the to-be-reviewed 100,000-document data collection containing English and Japanese. Then Rosette identified the sentences in the data collection.
The meaning of each exemplary email and sentence in the data collection was encoded in a mathematical representation using the word embeddings technique. Texts that are close in meaning will be numerically close in value.
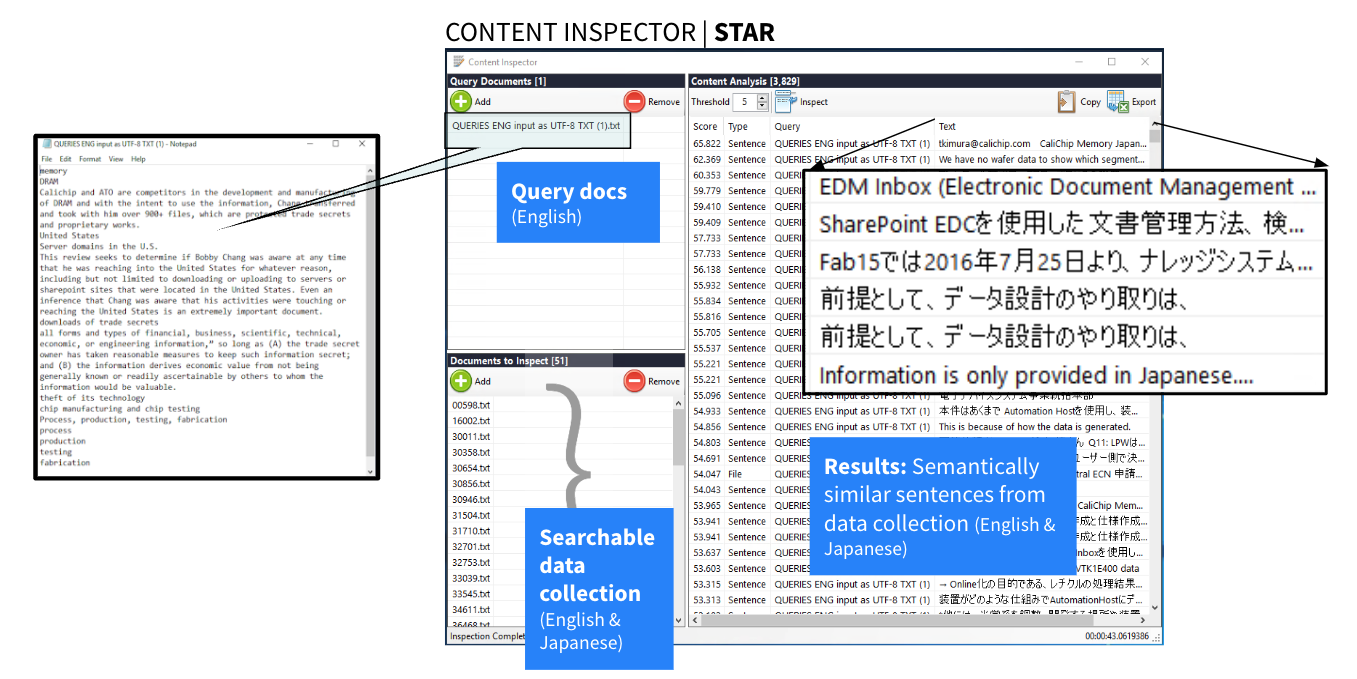
As illustrated in the above diagram, based on the query docs (exemplar emails), semantic search returned all sentences in the data collection that were semantically similar. From each sentence found, NLP-powered AI-extracted semantic keyphrases using part-of-speech tagging.
Then (as illustrated below) from the keyphrases, human staff extracted key terms from which to find more case-relevant keyphrases.
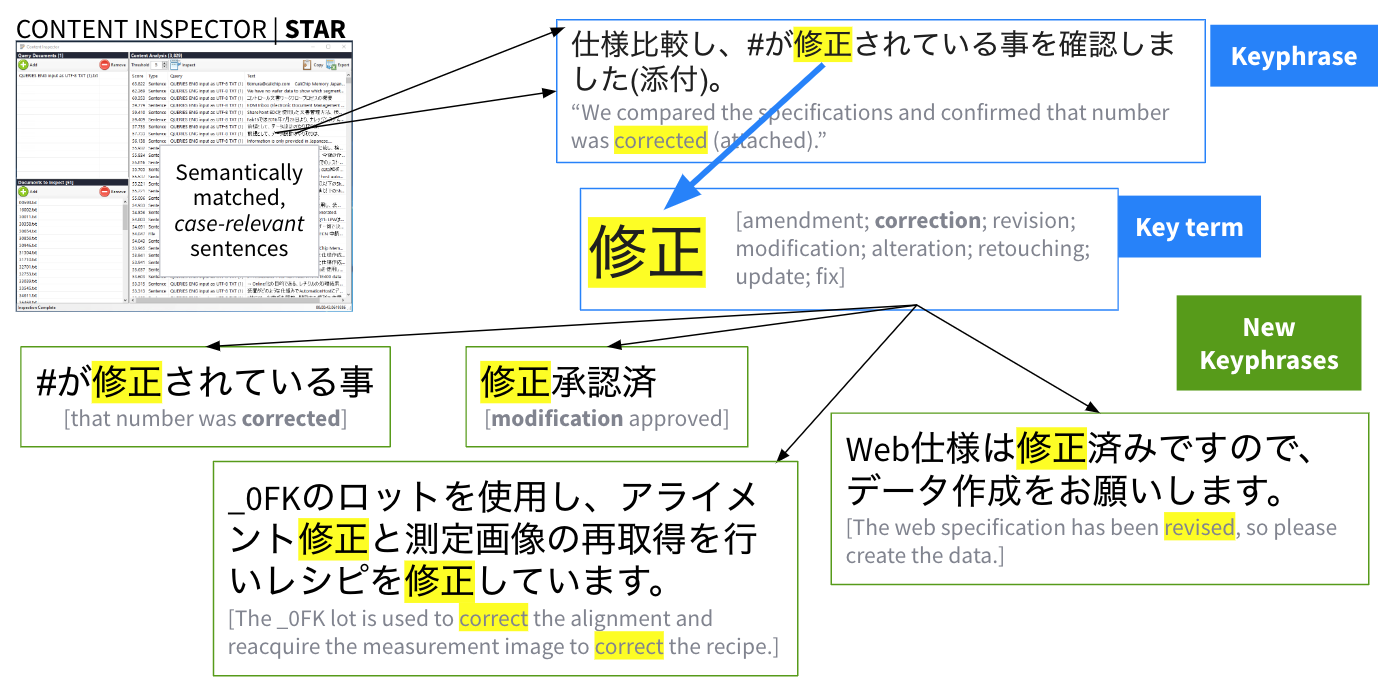
Case-relevant phrases to prioritize review
These keyphrases were used in two game-changing ways.
First, the semantic value of each keyphrase was compared to the semantic value of each unreviewed document in the data collection. In this case, there were about 2,000 case-relevant keyphrases used to rate the case relevancy of each file in the data collection.
Then, after adding up the scores, the highest scoring documents would be the top priority for human attorneys to review.

The new triage workflow (diagram below) combines text analytics in Rosette with the Ai Translate solution to create a powerful AI-based technology set and workflow tool — Semantic Translation Assisted Review (STAR). Leveraging these tools, the eDiscovery team improved the translation process while optimizing speed, cost, and accuracy.

Case-relevant phrases enhance machine translation
The next way phrases were used was for machine translation. The semantic keyphrases were translated by humans to enhance the glossary used by the the Ai Translate neural machine translation engine. There were two distinct improvements. First, garbled, partially translated sections became more intelligible machine translations.
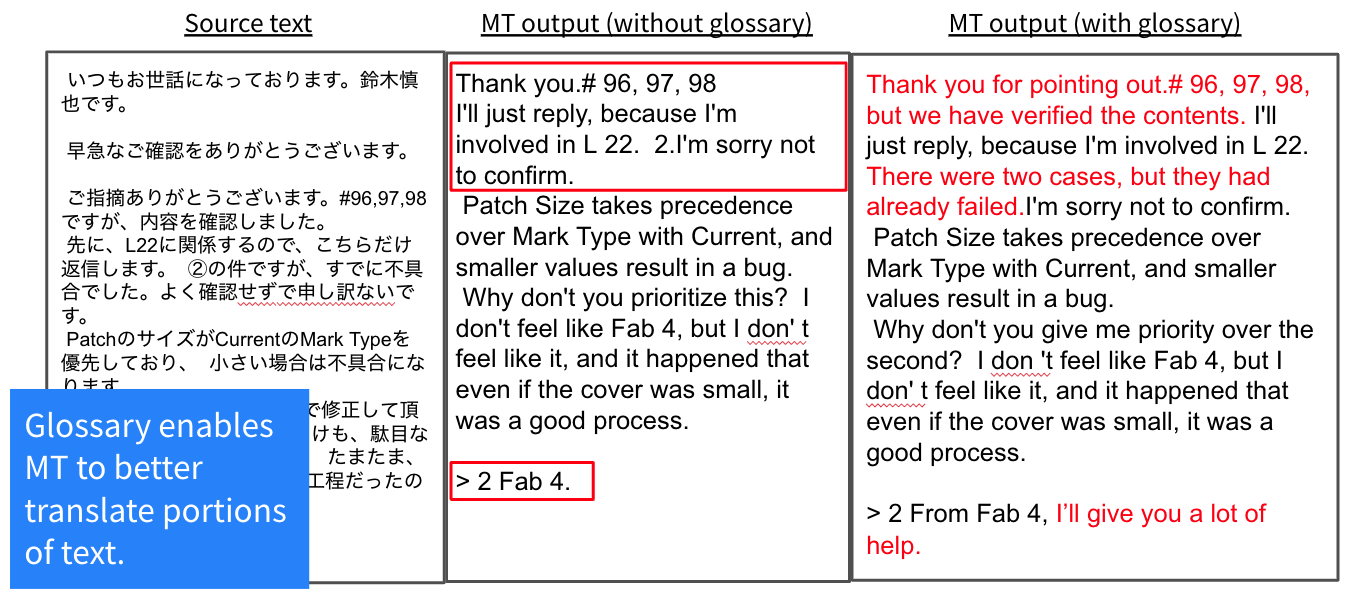
Portions of text that were partially translated became fully translated when the machine translation system leveraged the glossary.[/caption]
Second, in sections where the machine translation could not figure out a translation, it could do so with the glossary.

Skipped translations in the red boxed sections became machine translatable as shown in the corresponding yellow highlighted portions.[/caption]
With the glossary, translations overall became more nuanced, accurate, and consistent. Translations were further improved with named entity recognition from Rosette, which detected proper nouns (people, places, and organizations) and transliterated them. This step prevents machine translation from accidentally translating names literally. For example, when literally translated, the Japanese surname Azuma (東) becomes “east.”
As a result of these enhancements, attorneys who only speak English could review these Japanese documents well enough to determine if they should receive a fuller, human translation.
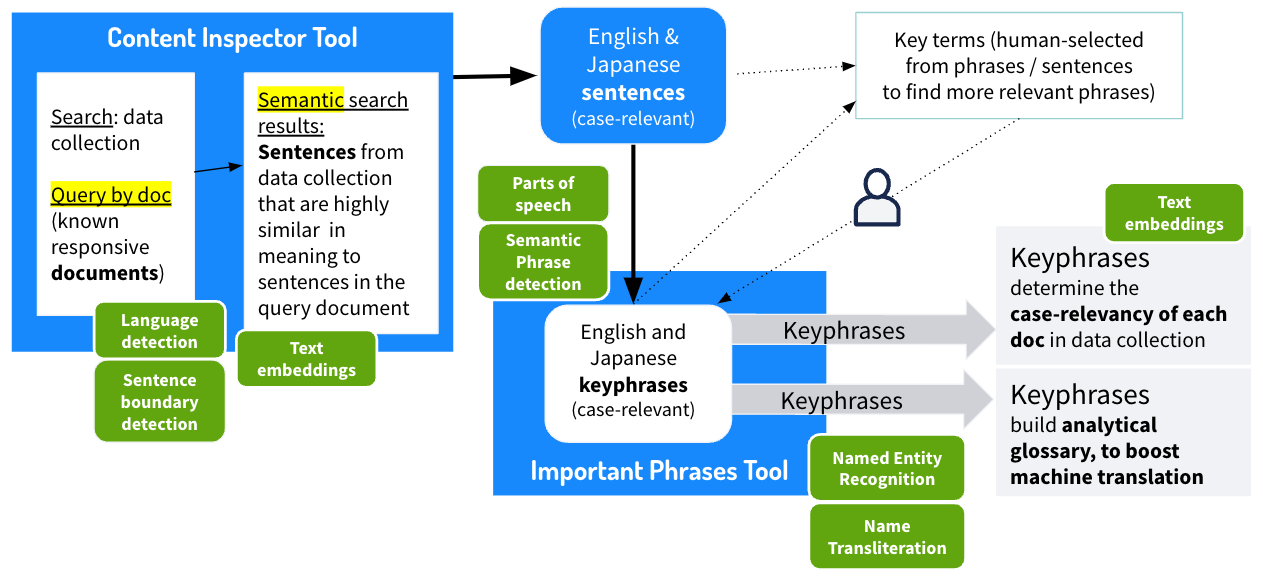
The new STAR workflow with NLP and machine translation enhancements.[/caption]
The impact of NLP and machine translation on multilingual eDiscovery
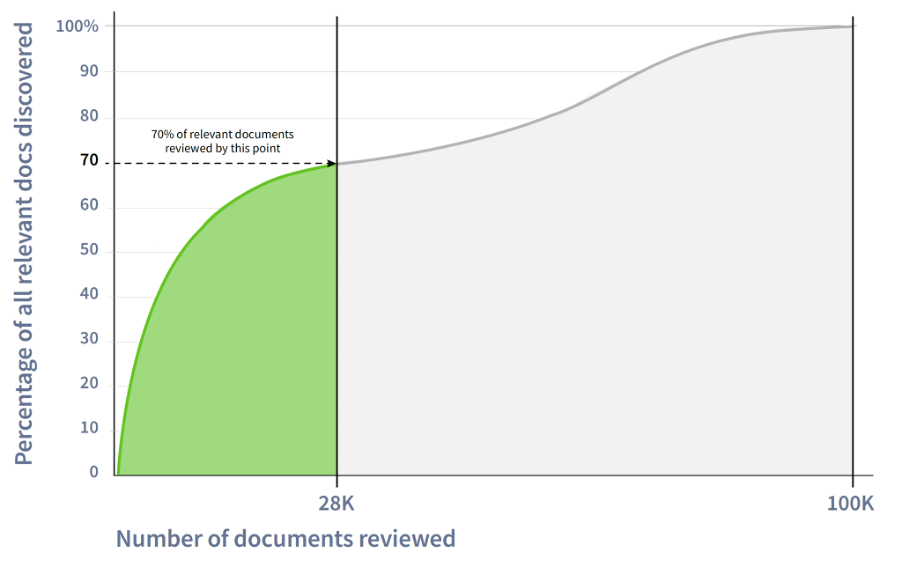
In this particular case, using STAR meant a review of 28% of the documents let attorneys see 70% of the relevant content.[/caption]
STAR prioritized the document review to start with the most content-rich, highly relevant files. This produced a huge savings in time and cost, and it also enhanced the quality of the review. From this data collection of 100,000 documents, a review of the top 28% of files captured 70% of relevant content. In brief, the legal team was able to defensibly downgrade 72,000 files, effectively increasing the review productivity by 151% and shrinking the multilingual eDiscovery timeline from months to weeks.
Conclusion
Businesses are still discovering ways to apply semantics to problems. This eDiscovery example was especially fruitful, and it is just one example of how semantics can effectively triage an overwhelming amount of data. Word-embedding NLP technology that enables semantic similarity comparisons reflect a significant step beyond keyword search methods, which have been regarded as “cutting edge” for decades. This, despite finding too much to feasibly review; but too little of what is relevant and ultimately dispositive of the case.
In this multilingual eDiscovery case, natural language processing with word embeddings and machine translation delivered:
- Data triage without relying on the help of bilingual attorneys
- Cross-lingual semantic search from English source text and queries
- Context-rich search that discovered unknown relevant content
- Prioritized document review, saving time and money
- Focused translation expenditures on useful content
Learn more about what word embeddings and AI-powered text analytics can do for you by scheduling a demonstration with Babel Street. For more information on Semantic Translation Assisted Review (STAR), you can download the STAR Fact Sheet or STAR White Paper.